RPA to Agentic AI: Challenges and Pathways for Lenders
February 10, 2025
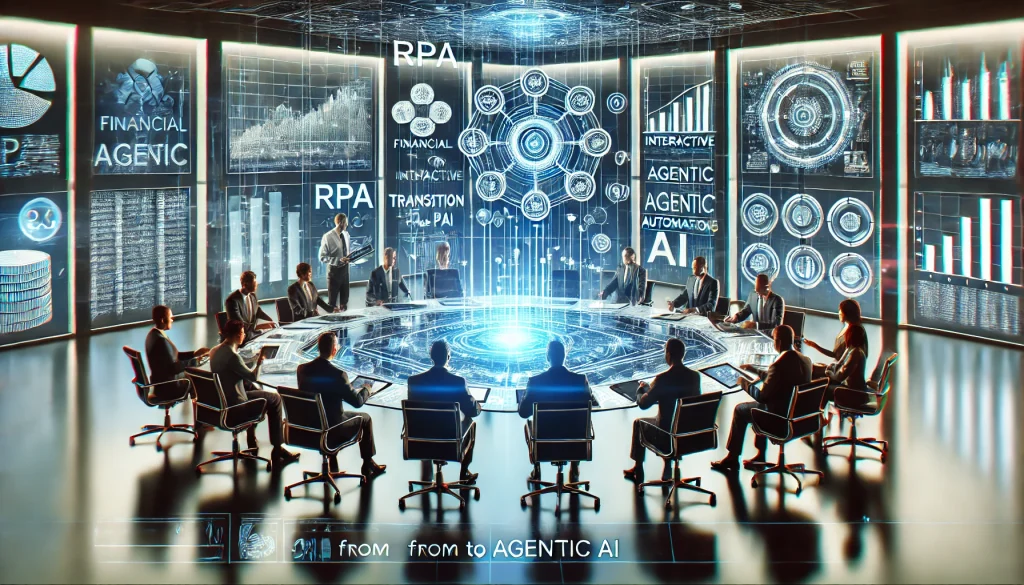
For years, mortgage lenders have relied on Robotic Process Automation (RPA) to streamline operations and reduce manual effort. While RPA has brought efficiency gains, the fact that it operates within predefined rule-based workflows limits its ability to handle complex decision-making. The emergence of Agentic AI, which can adapt dynamically, learn from past interactions, and make intelligent decisions, presents a new opportunity for lenders to go beyond automation into true operational transformation.
However, shifting from RPA to Agentic AI is not as simple as flipping a switch. In fact, it may require a wholesale abandonment of RPA in order to move forward. For CIOs, operations teams, and executives, this transition brings both opportunities and significant challenges that must be carefully navigated to maximize business value.
RPA vs. Agentic AI: Understanding the Shift
Before discussing the transition, it’s important to understand how Agentic AI differs from traditional RPA.
RPA automates tasks based on rigid, predefined rules. Oftentimes, RPA is literally just structured key-pressing the way a human might. At it’s best, RPA can organize more complex operations like API calls and integrations with other software programs. RPA requires structured input data and follows the same workflow every time, making it highly effective for repetitive processes. However, it lacks the ability to handle broad variability or adapt to exceptions, requiring human intervention when unexpected scenarios arise.
In contrast, Agentic AI introduces adaptability and intelligence to automations. Instead of executing a fixed set of instructions, it can interpret data in context, make informed decisions, and continuously improve its performance. This means AI-powered systems can process unstructured data (e.g. voice transcriptions, documents), detect anomalies, and even provide predictive insights, making automation more effective in complex mortgage workflows.
Challenges in Transitioning from RPA to Agentic AI
Despite its advantages, moving from an RPA-heavy infrastructure to AI-driven automation presents several hurdles for mortgage lenders.
One of the primary obstacles is technical debt and system integration. Many lenders have invested heavily in RPA solutions that are deeply embedded in their Loan Origination Systems (LOS), Customer Relationship Management (CRM), and servicing platforms. These systems were designed to work with predefined automation, not adaptive AI, creating challenges in compatibility. Upgrading legacy infrastructure without disrupting core operations requires careful planning, particularly when ensuring seamless data integration across various platforms.
Data complexity poses another significant challenge. AI thrives on structured and clean data, yet mortgage lenders often deal with incomplete, unstructured, or conflicting information from multiple sources. Many lenders rely on rigid, outdated validation rules that were designed for RPA, making them incompatible with AI-driven decision-making. To fully leverage Agentic AI, lenders must rethink their approach to data validation, compliance checks, and decision frameworks. Unlike RPA where these functions need to be “coded”, Agentic AI can be “taught.”
Regulatory and compliance risks further complicate AI adoption. The mortgage industry operates under strict guidelines, including Truth in Lending Act (TILA), Real Estate Settlement Procedures Act (RESPA), and Fair Lending Regulations. Any AI-driven automation must be able to explain its decision-making process, ensuring transparency and regulatory adherence. Unlike RPA, which follows explicit rules, AI models rely on probabilistic decision-making, making it essential to build compliance safeguards that allow for human oversight. Selecting the initial use cases on which to apply AI must adhere to the mantra that the lender is always ultimately responsible for the systems and data outcomes; They do not get the luxury to blame their technology or technology partners if AI takes an incorrect action or makes the wrong decision.
Beyond the technical aspects, organizational resistance to change can slow adoption. Employees accustomed to rule-based automation may hesitate to trust AI-driven decision-making, fearing job displacement or uncertainty in how AI systems will function in real-world scenarios. To mitigate resistance, executives must align AI initiatives with business goals, emphasizing how AI will enhance rather than replace human expertise. Training and change management programs are crucial in ensuring employees understand how to interact with and oversee AI-driven processes. Further, Agentic AI is always “learning”, so users will likely need to adapt towards providing feedback when AI gets something wrong.
Finally, cost and return on investment (ROI) concerns can act as a barrier to adoption. Many lenders have already made substantial investments in RPA, making it difficult to justify the additional expenditure required for AI transformation. While the long-term benefits of AI—such as increased efficiency, improved decision-making, and reduced compliance risks—are clear, the initial transition period may see productivity dips as workflows are restructured. Lenders must carefully evaluate the financial and operational impact before making large-scale AI investments.
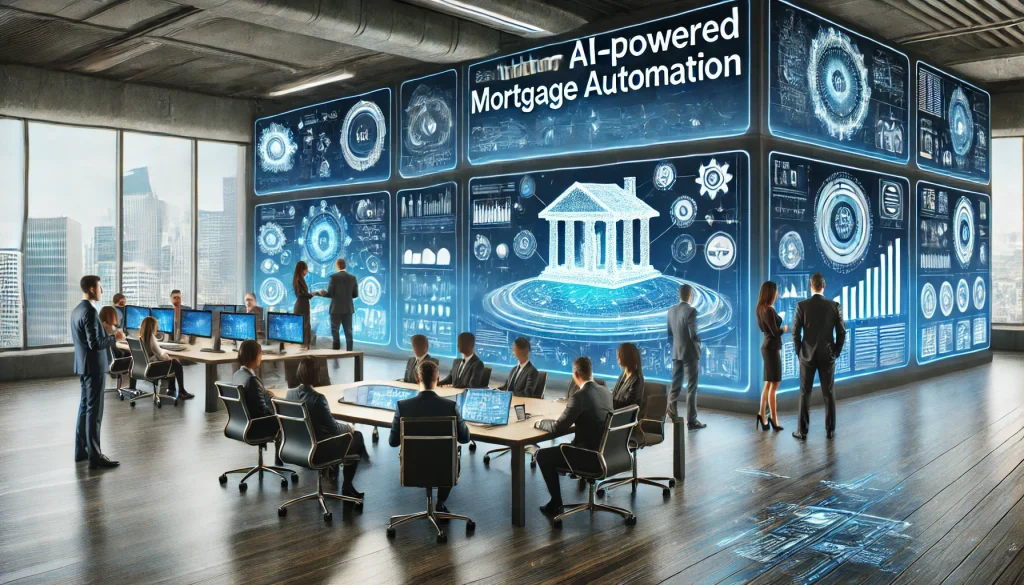
A Strategic Path Forward for Lenders
Despite these challenges, transitioning to Agentic AI can unlock significant efficiency gains and competitive advantages. The key to a smooth transition lies in phased implementation rather than an abrupt replacement of existing automation systems.
Step 1: Identify High-Impact Use Cases
The best way to start is by identifying areas where Agentic AI can provide immediate ROI. Key opportunities include document classification and validation (e.g., Vallia DocFlow), automated underwriting enhancements (e.g., Vallia AUS Sandbox), and AI-driven customer engagement (e.g., Vallia AI Chat for Loan Officers). As noted earlier, it is preferential that the first use cases not be focused on areas where an incorrect decision or action will have a severe negative impact.
Step 2: Implement Hybrid Automation
Rather than replacing RPA outright, lenders should first augment RPA with AI components. AI can be used to detect anomalies, enhance decision-making, and handle complex exceptions, while RPA continues to automate repetitive tasks. See our recent Case Study on how we approached this problem.
Step 3: Focus on Data Optimization
Since AI effectiveness depends on high-quality data, lenders must invest in AI-driven data validation tools that clean and standardize information before it is processed. Intelligent document processing (IDP) solutions like Vallia DocFlow ensure compliance and reduce errors and can be leveraged to feed other document-heavy process AIs with quality data (e.g. disclosures, servicer/investor integrations).
Step 4: Address Compliance & Transparency
To meet regulatory requirements, AI systems must be designed with explainability in mind. Implementing human-in-the-loop AI models ensures compliance alignment and builds trust among regulatory bodies. Tools like Vallia AUS Sandbox are structured to leverage AI as a coach rather than a standalone agent. This allows a safe transition into accepting the technology.
Step 5: Gradual Workforce Integration
Lenders must proactively prepare their workforce for AI adoption. Training programs should emphasize collaboration between humans and AI, demonstrating how AI can augment employees rather than replace them. “AI” conjures-up scary notions for many workers but the reality is that in the near-term, AI is just the latest power tool that can make them more productive. Pilot programs can help ease employees into new workflows, allowing for gradual adoption before full-scale rollout.
Step 6: Monitor & Iterate
AI is not a one-and-done solution; it requires continuous monitoring and refinement. By tracking AI performance and business impact, lenders can adjust workflows as needed and ensure AI models remain aligned with business objectives. User feedback and real-time system monitoring should be built into the transition strategy.
Conclusion
Shifting from RPA to Agentic AI is a strategic evolution rather than a simple technology upgrade. The potential benefits—greater efficiency, smarter decision-making, and an enhanced borrower experience—are undeniable, but achieving them requires overcoming challenges related to legacy infrastructure, data complexity, regulatory compliance, workforce adaptation, and cost considerations.
By taking a phased and strategic approach, lenders can gradually integrate AI-driven capabilities without disrupting existing operations. Brimma Tech’s Vallia AI solutions provide a clear path for lenders to modernize their technology stack while leveraging their current investments.
For CIOs and executives, the decision is no longer about whether to adopt AI, but rather how soon they can start unlocking its potential.
Interested in AI-driven mortgage automation?
🔗 Contact Brimma Tech to explore how our Vallia AI ecosystem can future-proof your lending operations. 🚀