AI Before RPA – A Case Study
February 10, 2025
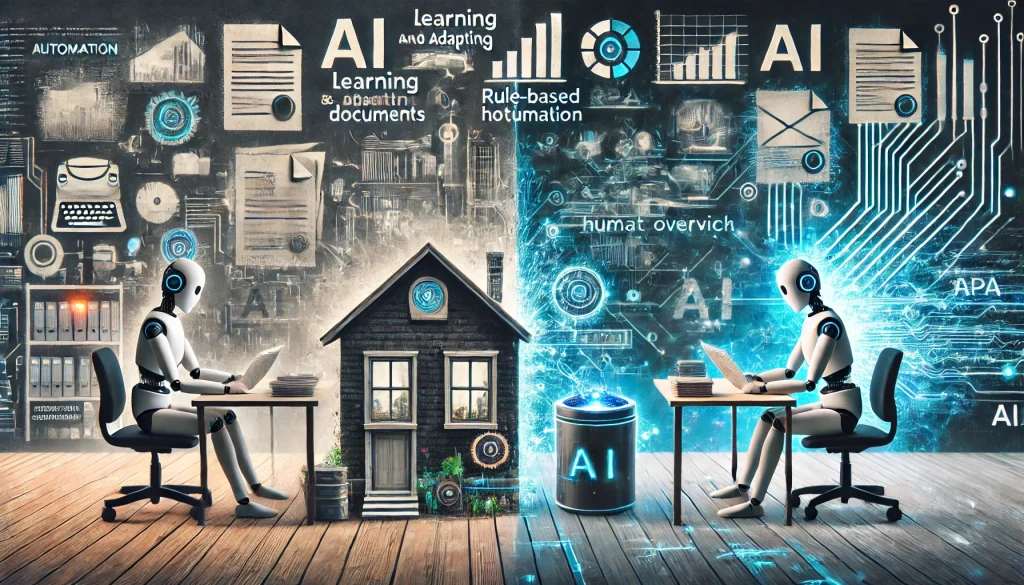
A small but ambitious mortgage lender found itself at a familiar crossroads—manual processes were consuming valuable time, limiting scalability, and increasing operational costs. With minimal automation in place, they needed a solution that would deliver quick and measurable benefits without requiring an extensive upfront investment. Rather than taking a traditional path with low-cost Robotic Process Automation (RPA) for specific tasks like disclosures, MERS, or ULDD, the lender explored a more advanced, AI-driven approach that would yield greater efficiency right from the start.
The Challenge: Finding the Fastest Path to Value
Like many smaller lenders, this company relied heavily on manual processes for document handling, which resulted in delays, human errors, and inefficiencies in loan processing. While RPA could automate some isolated tasks, the lender sought a broader solution that would streamline workflows without requiring constant updates to automation scripts. Their leadership team needed a system that aligned costs with per-loan pricing, making it easy to measure ROI and justify future expansion.
The Solution: AI-Powered Document Classification
After reviewing Brimma’s suite of automation solutions, the lender decided that an AI-powered document classification subsystem would provide the quickest and most impactful benefits. By leveraging AI to organize and index incoming documents, the lender could eliminate one of the most time-consuming aspects of loan processing. The technology team worked closely with Brimma’s implementation specialists, ensuring a tight feedback loop and rapid deployment. Within just two weeks, the system was production-ready for an initial set of low-risk use cases.
At first, the lender took a conservative approach, choosing not to allow AI to make direct changes to their Loan Origination System (LOS). Instead, they deployed a side-by-side validation dashboard, allowing staff to compare AI-generated classifications with their existing manual indexing process. This cautious strategy helped build confidence in AI’s accuracy while giving the team time to adjust their workflows.
Results: From Indexing to Intelligent Automation
After just one week of running the AI-driven classification system in parallel, the lender saw consistent accuracy and time savings. With initial success in indexing, they took the next step—allowing AI to update the LOS when document matches were verified. Over time, they expanded its role further, enabling the system to route documents for human review when validation rules failed, ensuring compliance without disrupting existing operations.
By taking an incremental, low-risk approach, the lender not only achieved immediate efficiency gains but also created a clear roadmap for future automation expansion. Instead of rushing into AI with a risky, all-or-nothing approach, they methodically extended its impact in a way that supported their existing workflows.
Key to Success: A Commitment to Execution
One of the defining factors in the lender’s success was the strong commitment from internal stakeholders. Rather than allowing delays or overcomplicating the project, leadership set clear expectations that the team would make this effort work. This mindset ensured that every challenge was met with a problem-solving approach, rather than an excuse to slow down progress.
Future Expansion: A Scalable Automation Strategy
With a strong foundation in place, the lender now has a measured and scalable path forward. The AI-powered system is already handling document classification and validation, but its potential extends to broader mortgage automation tasks, from compliance checks to deeper integration with the LOS. Because of the gradual and methodical rollout, the lender can continue expanding automation without disrupting existing operations, ensuring a seamless transition to a more intelligent lending process.
Conclusion
By skipping traditional RPA and embracing AI-driven document classification, this lender unlocked efficiency gains faster than expected, with a direct and measurable return on investment. Their experience demonstrates that even smaller lenders—when equipped with the right technology and an execution-focused mindset—can achieve big automation wins in a short timeframe.
Perhaps most notably, this AI-driven approach has redefined the lender’s automation strategy. Instead of implementing multiple isolated RPA solutions for individual tasks like indexing disclosures, validating MERS registrations, or processing ULDD submissions, they now have a single AI-powered core that can handle these functions and more. What began as an effort to automate document classification has expanded into a broader automation framework, replacing many of the manual tasks that would have traditionally required RPA bots.
With AI now serving as the foundation for all document-centric automation, the lender has a clear path forward—one that eliminates the complexity of managing multiple RPA scripts and instead leverages a unified, intelligent system that can continuously learn, adapt, and scale. By methodically expanding the AI’s capabilities, they are ensuring that future automation efforts are not only efficient but also future-proof, setting the stage for greater innovation and long-term success.